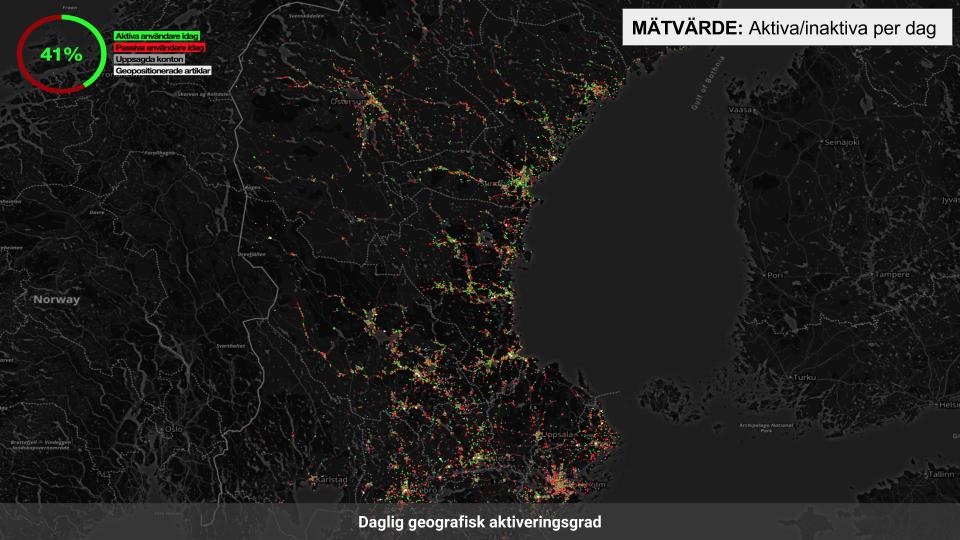
This article was originally published in Medievärlden Premium.
Published with permission.
Hyperlocal Antichurn, a Scandinavian Business Case
partner insights
Being local is one of the key success factors for our partners, though their industry expertise is also relevant for an international audience.
In the Partner Insights series we share their stories.
The third largest media company in Sweden, Mittmedia with 23 daily local newspapers and a circ. of almost 300.000, are battling reader churn with the use of interactivce maps.
In our Scandinavian markets, much of the focus in 2017 was on conversion, for 2018 activities aimed at minimizing churn have emerged as equally, or maybe even more, important than conversion.
A current use-case from Mittmedia presented by Medievärlden Premium (link below) gives some understanding of how they are dealing with the issue.
At the end of 2017 Mittmedia had 50.000 digital subscribers. And need to convert 1.200 new “plus” subscribers/week in order to reach their 2018 target of 100.000.
But even more important than conversion is to reduce churn. Churned subscribers are much harder to convert than users that have never subscribed before.
One of the most efficient measures to reduce churn is to increase the activity among users. For this purpose, Mittmedia is constantly monitoring user activity over the last 14 days.
During Nov-Dec 2017, 50% of all users were active every day or every second day.
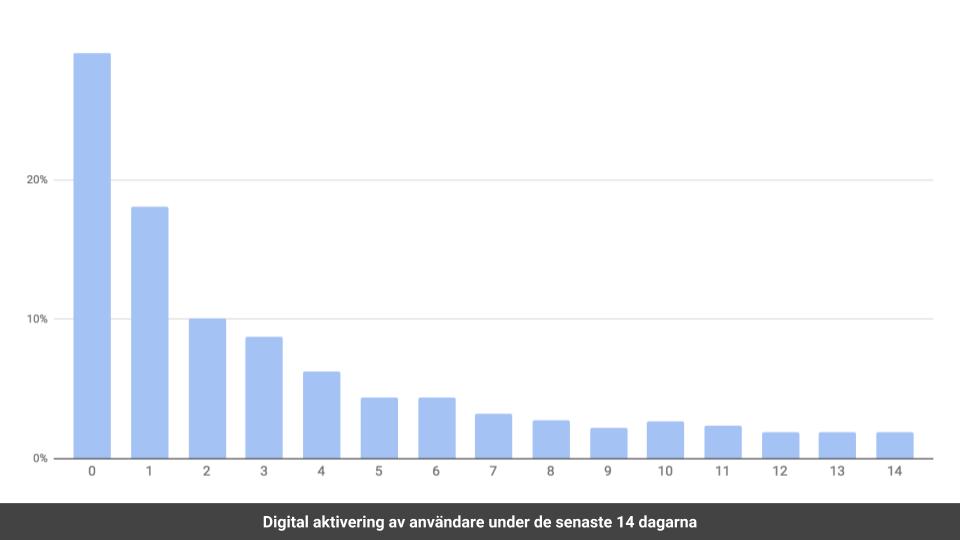
Digital activation of users during the last 14 days
Mittmedia is focusing on activities for these 50%. For the other groups, there are many opportunities for personalization. But the less days their users utilize Mittmedia’s digital products, the fewer opportunities there are to reach them.
Examples of personalization: New Plus-customers that have showed an interest in the Business-section and that have not logged in for the last 14 days, automatically get a newsletter/news digest summarizing Business news for the last 14 days as they log in again.
There is a three-step personalization of content:
- Topics of interest to the user
- Topics that are of interest to other users based in the geographical proximity
- A smart mix of 1+2
One example of 2 might be a sports event that create a huge interest in a certain geography/part of town.
In addition to the packaging of content, customer-oriented activities and campaigns are also very important. For this customer segmentation is essential. Mittmedia have segmented their customers based on how often they log in:
- Day 0-1 (50% of customers) Packaging of content
- Day 2-6 (30% of customers) “Display value”, Local campaigns
- Day 7-14 (20% of customers) E-mail campaigns, Reach campaigns
In Category 2-6 days, the “Display value” activities can include showing the customers content that they might be interested in (based on data) but not currently are using (also based on data). For example, a customer that is interested in Ice-hockey, but that is not using the Mittmedia Hockeypulse site or Hockeypulse app.
In such cases Mittmedia might run a campaign with the message: “Do you know that signing up for a Plus -subscription will also give you access to Hockeyplus?”
In the last and most critical category (7-14 days), personalized newsletters play a big role. Compiling a digest of content that is likely to be of the interest for the customer.
In addition to the segmentation above, customers are also placed in four different groups depending on type of subscription: Total-customer, Weekend-customer, Digital-customer and Plus-customer.
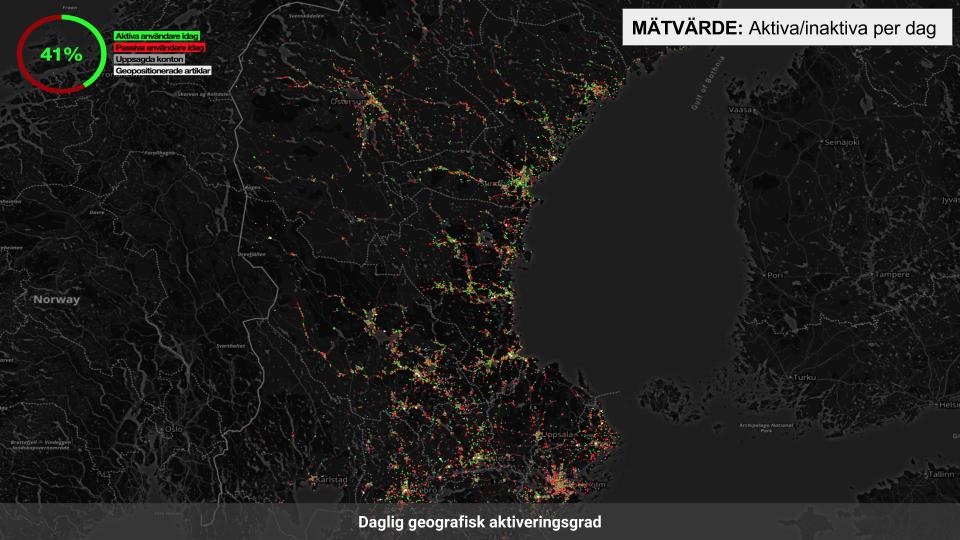
Daily level of activation by geography.
Mittmedia have developed a powerful tool to counter churn. It is a map where the editors can follow usage from individual households live. Each dot is a household, a grey dot indicates a churned household, a red dot indicates inactivity for the last 24 hrs while a green dot indicates activity within the last 24 hours. Geopositioned articles are also plotted as white dots on the map.
If there are a lot of red dots in an area, a direct activity can be launched, e.g. a push-notification in the area. If staff has a choice between covering events in different areas, they might prioritize an area with a lot of red dots. Should there be a lot of grey dots, it might be an indication that more editorial coverage is needed in this area.
The use of this map has proven effective as regards both activation and churn. In October the activation was never above 60%. As soon as the map was implemented by end of October, activation immediately raised to 70% and above, and has stayed there since.
As mentioned earlier, a churned subscriber is harder to convert than someone who has never subscribed. In analogue with this, a campaign that attracts a large number of new customers might actually do more harm than good. One example of this is when Östersund FK played the Europa League and Mittmedia had the rights to the games and covered these for their “plus” subscribers. Over 20.000 customers saw the soccer games in Greece and a huge number of conversions took place, but then many of these churned (and will thus be harder to win back).
When spending resources to win new customers, one of the most important factor is that these measures must be long-term. If not, you are only moving customers to the group that is the hardest to convert. Meaning that you will have “destroyed” the customer base. The customer base must be grown with a long-term focus and in the home market/territory.
One aspect of the anti-churn activity might be to identify interesting content in the summer for subscribers to Bandypuls (Bandy is a wintersport). Which content this might be, should be possible to determine by analyzing data. If you dive deep into the available data, you will see how the different “plus”-services are used. As an example, Bandypulse yields more users than Hockeypuls, while the daily usage is about the same. This means that Hockeupuls-users, to a greater extent are already Mittmedia-subscribers. With this information the different services can be utilized in the most efficient way.
The personalization of topics is achieved through machine learning using two separate processes:
- For existing users, based on their logged-in activity. Machine learning is done by using this data.
- For new users. The findings from #1 above, is applied in order to place the new users in the right cluster
The clusters are created through machine-learning and new users are distributed among these clusters using machine-learning. NB. The clusters are created without human interaction, only the naming of the cluster is a job for humans.